Machine Learning In Finance: 12 Essential Applications
The impact of machine learning on finance is significant. Thanks to this technology, financial institutions are now equipped to make efficient decisions. Through the analysis of data sets, machine learning algorithms identify patterns, forecast trends and automate processes. It’s akin to having a tool that predicts the future based on data.
Banks, for example, leverage machine learning to detect questionable activities, personalise customer interactions and enhance risk management practices. Have you ever wondered how your bank always seems to know which offers will catch your attention? Chances are high that machine learning plays a role in this process.
This piece delves into the applications of machine learning within the finance sector. It provides examples and suggests actionable steps for implementation. Consider it as a guide to optimising operations, reducing expenses and fostering creativity within your organisation.
Table of Contents
Download your free “Gamification Guide”
Download the Mambo Gamification Guide for detailed insights and actionable strategies to enhance engagement and performance.
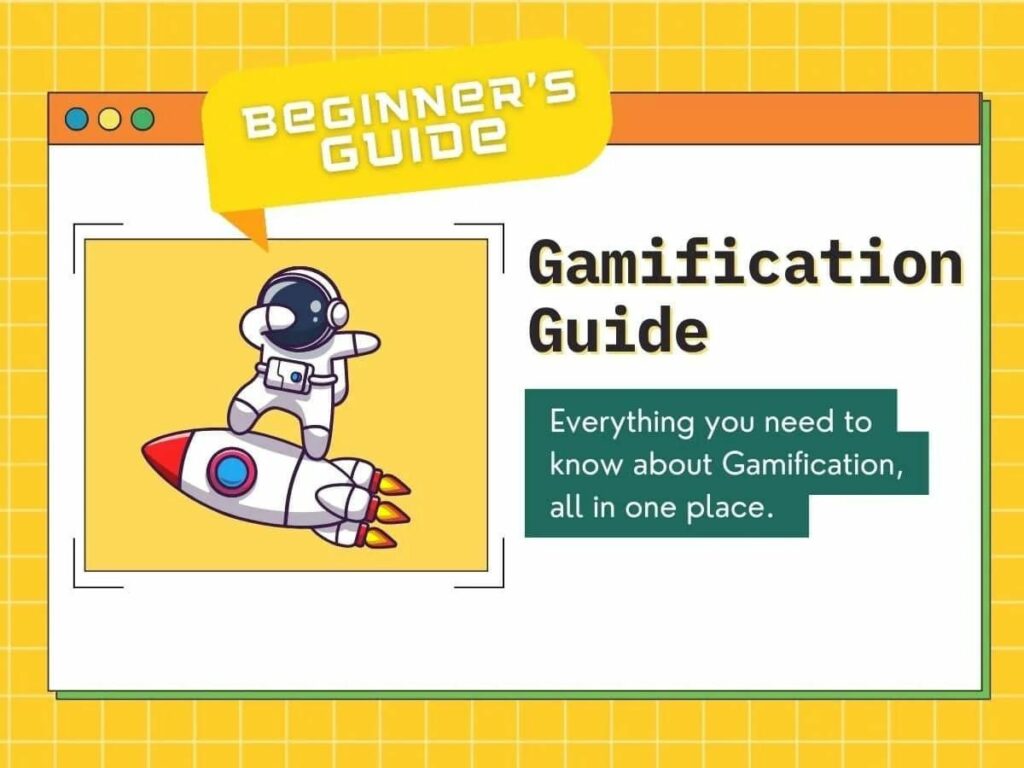
What does machine learning entail?
Machine learning is all about teaching computers to understand data. That is, they can make their own decisions without needing step-by-step instructions for every single task. Remember when Arthur Samuel dropped his checkers-playing program in the ’50s? Do you recall how that particular program kept improving over time through constant practice? That showed a glimpse into the capabilities of machine learning.
Consider it allowing your computer to spot trends and make predictions, much like a seasoned stockbroker. These models iteratively learn and refine their accuracy by crunching through massive datasets. Unlike old-school programming with rigid rules, machine learning systems evolve and adapt based on their experiences.
The rise of machine learning in finance
Machine learning, which dates back to the 1950s, began making waves in the finance world in the early 2000s. Do you recall when financial organisations were overwhelmed with data? They needed a lifeline, and machine learning threw them a rope. This technology goes beyond processing numbers. It has the ability to detect patterns and trends that may elude an analyst.
Think about it: predicting market shifts and assessing credit risk — machine learning makes these tasks almost second nature for financial pros. It’s like having a co-pilot for navigating through today’s markets. Nevertheless, challenges do arise. Sometimes, the data can be tricky, and the models need constant tweaking.
Rapid adoption and proven results
According to a Deloitte report, 67% of financial institutions have already woven it into their operations. Why the rush? Honestly? The perks are just too great to ignore.
Let’s looks at JPMorgan Chase, PayPal and Robinhood. These giants are diving headfirst into the machine learning pool, splashing out on tech, cutting fraud and boosting customer satisfaction. It’s like they’ve found the secret sauce.
Speaking of results, machine learning is crushing traditional methods. Imagine this: a 20-30% improvement in predicting credit risk and a staggering 40% boost in stock price forecasting accuracy. These numbers aren’t just stats; they’re rewriting the entire rulebook.
12 essential applications of machine learning in finance
In this section, we’ll explore the essential applications of machine learning in finance and guide you on implementing these technologies to maximize your business potential.
#1. Fraud detection and prevention
Fraud detection and prevention in finance? It’s all about spotting and stopping sneaky activities before they cause damage. Picture this: advanced tech like machine learning sifting through massive datasets, hunting down anomalies that scream “fraud.”
Financial institutions deploy these robust systems to monitor transactions, ensuring everything’s legit. They use pattern recognition, real-time analysis, and anomaly detection.
These machine-learning algorithms are like eagle-eyed detectives, separating the good guys from the bad guys. Trust me, this tech is revolutionising finance, safeguarding assets, cutting risks, and earning customer trust.
Real-world examples and case studies
Take PayPal, for instance. They’re a prime example of using machine learning to sniff out fraud. Every day, they monitor and analyze millions of transactions. When something fishy pops up, their system flags it, allowing for a quick response. This way, PayPal ensures transaction safety and wins customer trust.
Another example? JPMorgan Chase. They rely on machine learning algorithms to spot unusual transaction patterns. Training these algorithms on historical data helps them catch fraud attempts early, saving big bucks. These stories show how powerful machine learning is in boosting financial security and nipping fraud in the bud.
Key benefits
- Enhanced security: Accurately identifies suspicious activities, leading to fewer monetary losses.
- Boosted productivity: Quicker transaction handling through reduction of reliance on manual verifications.
- Decreased false alarms: Time and resources saved by cutting down on incorrect fraud notifications.
- Enhanced client contentment: Accurate fraud identification upholds customer loyalty.
- Substantial financial benefits: Mitigating fraud risks leads to savings worth millions.
- Predictive abilities: Allows banks to proactively address challenges.
- Overall benefit: It creates a safer and more reliable financial system.
Enhancing fraud prevention with gamification
Adding gamification elements like points, levels, and rewards can really boost fraud prevention training in finance. Why? It makes learning fun and interactive. Imagine earning rewards while learning crucial skills. This approach keeps users engaged and motivated.
Here’s how some elements can be incorporated:
Points system
- Accumulation: Employees earn points for completing modules, answering quiz questions correctly, and participating in discussions. For example, a training module on identifying phishing emails could award points for each correctly identified example.
- Leaderboards: Display leaderboards that show top point earners, motivating employees to compete and engage more thoroughly with the content. For instance, a monthly leaderboard can highlight those who have completed the most training modules or scored highest on fraud detection quizzes.
Levels
- Progression: Training programs can be structured into levels where employees progress as they complete modules and earn points. For example, starting at beginner levels with basic concepts like understanding typical fraud schemes and advancing to more complex levels involving sophisticated detection techniques and case studies.
- Unlocking content: Higher levels can unlock advanced content and access exclusive webinars or practical exercises. This encourages continuous learning and rewards those committed to enhancing their skills.
Rewards
- Incentives: Offer tangible or intangible rewards. These could be certificates, badges, recognition in company communications or even minimal monetary bonuses. Let’s say employees finish a fraud prevention course. They could earn a “Fraud Prevention Specialist” badge. That would make them feel “seen” and appreciated. It would also show their dedication and expertise.
- Recognition: Take a moment during team meetings to give a shoutout to top performers. Why not mention them in newsletters, too? It’s an excellent way to boost morale and get everyone more engaged. Plus, who doesn’t enjoy a little recognition?
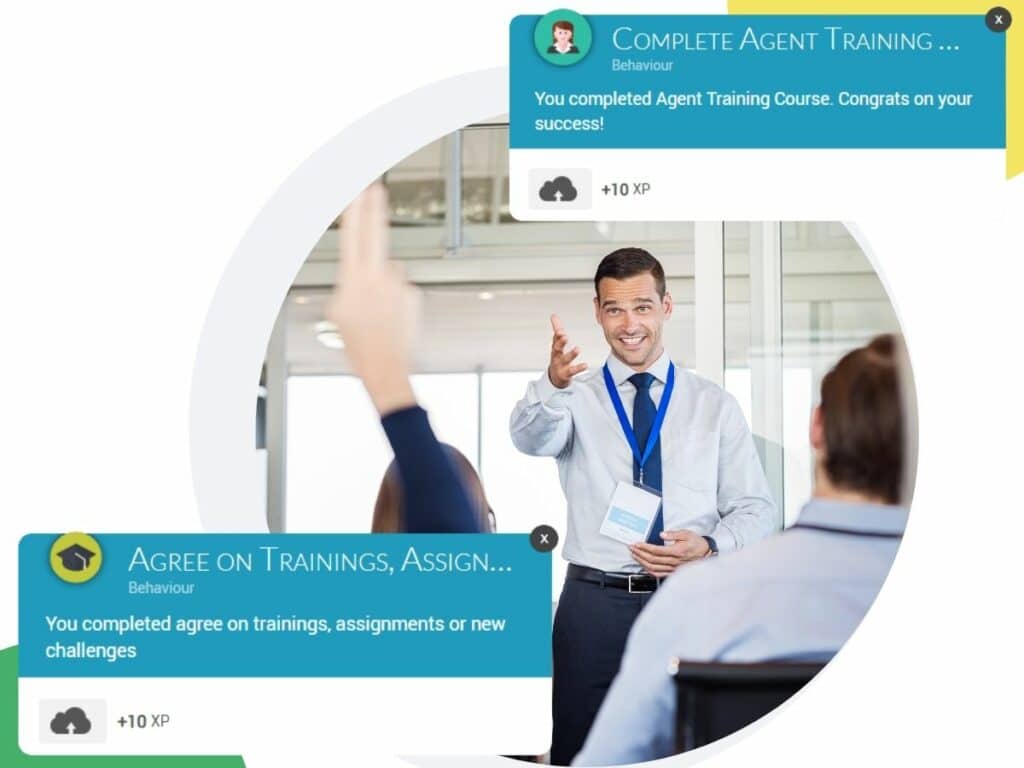
#2. Algorithmic Trading
Algorithmic trading, or algo trading, is like having a super brain. Imagine a computer making lightning-fast decisions for you. It uses algorithms and machine learning to sift through tons of data. Think of it as your tireless assistant, forever finding the best deals based on set rules.
You know how humans can get emotional or make mistakes? Algo trading aims to cut that out, maximizing returns while minimizing risks. It’s not foolproof, though. Sometimes, the market can behave unpredictably, and even the best algorithms can stumble.
I’ve seen traders swear by it, saying it’s revolutionised their strategy. But, they also warn about over-reliance on technology. Balancing human intuition with machine precision is key.
Still, algo trading is transforming the financial sector. It’s allowing traders to manage intricate strategies that would be nearly impossible to manage manually.
Real-world examples and case studies
For instance, Renaissance Technologies has developed super-secretive algorithms that help them make huge profits. Citadel LLC, meanwhile, takes advantage of these algorithms to trade and predict market movements with impressive accuracy.
Goldman Sachs has also jumped on the bandwagon, utilising algo trading to streamline its market-making operations. The first step taken by these financial giants typically involve developing algorithms based on mountains of historical data. They will then test them rigorously and then deploy them.
The results? Faster trade execution, lower transaction costs, and more accurate market predictions. These real-world cases show just how transformative algo trading can be.
Key benefits
- Speed: Executes trades faster than any human, ensuring the best prices.
- Consistency: Eliminates emotional biases, leading to more consistent trading outcomes.
- Reliability: Follows strict, data-driven rules, making it dependable and accurate.
- Strategy Optimization: Allows for testing on historical data to fine-tune trading strategies.
- Efficiency: Processes massive amounts of data quickly, reducing transaction costs and minimizing market impact.
- Competitiveness: Precision and speed make it essential for staying competitive in the finance industry.
#3. Personalised banking and finance
Imagine your bank knowing precisely what you need before you even ask. That’s the magic of personalised banking and finance using machine learning.
By analysing heaps of data—like your spending habits and financial goals—banks can offer tailored recommendations just for you. It’s like having a financial advisor who knows you inside out, except it’s driven by algorithms.
The core idea here is making decisions based on real-time data, which makes financial services more relevant and efficient. Banks get to understand their clients better, offering precise advice and solutions.
Real-world examples and case studies
Take Capital One, for example. They leverage machine learning to sift through transaction data and send personalised savings tips through their app. It’s essentially about gathering data, training models and deploying insights on the fly.
Wells Fargo has joined the fray, too, using AI to customise customer interactions and financial advice. The results? Happier customers and wiser financial decisions.
Key benefits
- Increased customer engagement: Enhances interaction and connection with customers.
- Improved financial results: Delivers better financial outcomes for both banks and customers.
- Timely and relevant advice: Machine learning provides advice that boosts customer satisfaction.
- Smarter decisions: Data analysis leads to improved efficiency and reduced costs.
- Tailored financial goals: Helps customers achieve specific financial objectives, such as saving more or earning better returns.
- Actionable insights: Turns vast amounts of data into useful information, building deeper trust between customers and banks.
- Enhanced quality of services: Elevates the overall quality of financial services to a new level.
#4. Credit scoring and risk management
Credit scoring and risk management are critical in the finance industry. Credit scoring rates individuals based on credit history and repayment patterns, while risk management identifies and mitigates financial risks.
Advanced analytics and machine learning have taken these processes to the next level. They enhance them by analysing vast amounts of data to detect patterns traditional methods might miss. This technology helps lenders evaluate loan applicants and manage portfolio risks, improving financial decision-making and economic stability.
By integrating diverse data, these systems provide a clearer picture of borrower risk profiles, leading to smarter lending decisions.
Real-world examples and case studies
Banks and financial institutions are all in on machine learning for credit scoring and risk management.
Take JPMorgan Chase, for instance—they use AI models to predict loan defaults. Their system dives into payment history and spending patterns, providing real-time credit evaluations.
Another cool example is ZestFinance, which uses non-traditional data like utility payments to predict defaults. This approach has upped their accuracy considerably.
Key benefits
- Enhanced accuracy: Identifies patterns traditional methods miss, leading to more precise credit assessments.
- Real-time analysis: Offers quicker and smarter decision-making processes necessary in a fast-paced market.
- Increased efficiency: Automates data analysis, markedly decreasing manual workload.
- Improved risk detection: Helps spot risks and make swift decisions.
- Reliable operations: Promotes more reliable and efficient financial operations.
- Continuous improvement: Encourages ongoing refinement and caution to maintain system effectiveness.
#5. Churn prediction
Churn prediction is akin to peeking into the future for financial institutions. It uses machine learning to figure out which customers might jump ship. By analyzing past data—like transaction histories and demographics—it identifies who’s likely to leave.
Think of it as noticing patterns before they become problems, allowing banks to step in and fix things. This is super important in finance, where keeping customers happy is the name of the game. With machine learning, vast amounts of data are processed to make accurate predictions.
The real magic? Reducing client losses and improving how banks manage relationships. They can address issues before it’s too late by spotting potential churn.
Real-world examples and case studies
Financial institutions, big banks and local credit unions are all over churn prediction. Picture a bank that sees a customer’s account activity dropping. With a churn model, they flag this person as a flight risk.
What’s next? Personalised incentives to keep them around. Case studies from top banks show this works wonders—they report better retention and happier customers. Usually, it means plugging machine learning models into existing data systems.
The results? Lower churn rates and boosted loyalty, proving that this tech works.
Key benefits
- Early risk identification: Detects customers at risk of leaving, allowing proactive action.
- Deeper customer insights: Provides a more accurate assessment of customer behaviour that leads to more personalised services.
- Cost savings: Keeping existing clients is more cost-effective than pursuing new ones.
- Enhanced decision-making: Upgrades decision-making methods by leveraging data-driven insights.
- Reduced customer attrition: Deals with client churn to help cultivate a more loyal customer base.
- Enhanced engagement: Contributes to a broader strategy for keeping customers engaged and satisfied.
#6. Loan approval
Loan approval is basically about determining if someone can repay the loan. It functions as a financial background check where credit scores, income, and job history are all reviewed.
Back in the day, this whole process comprised manual and tedious work. It was also prone to errors. Imagine sifting through piles of paperwork. But now, with the magic of machine learning, we’ve gotten a lot more efficient.
Algorithms now handle most of the heavy lifting, speeding up decisions and getting more accurate results. This shift is crucial; after all, lenders need to make sure loans get repaid. Embracing machine learning means better risk management and less bias—pretty much a win-win for everyone involved.
Real-world examples and case studies
Let’s look at some real-world stories where machine learning really shines in finance. Take a big bank that implemented machine learning for evaluating loan applications. Picture an army of algorithms sifting through data to predict who’s likely to repay.
Another case? A fintech startup using advanced models for on-the-spot loan decisions. These tech-savvy approaches have sped things up and slashed default rates. Banks and fintech companies report not just faster approvals but also happier customers.
Key benefits
- Faster decisions: Provides loan approval answers in minutes instead of days.
- Increased accuracy: Reduces defaults, leading to higher satisfaction for all parties.
- Enhanced fairness: Minimizes human bias, resulting in more equitable loan assessments.
- Better risk management: Improves the ability to manage risk effectively.
- Smoother operations: Streamlines the loan approval process, reducing delays and human errors.
- Efficient and fair process: Machine learning makes loan approvals more efficient and just.
#7. Bankruptcy prediction
Bankruptcy prediction uses data analysis to determine if a company might fail, helping financial firms assess creditworthiness and manage risks. Models like the Altman Z-score and advanced machine learning detect risk patterns, aiding informed decisions.
Financial institutions rely on these predictions to evaluate loans, investments, and market stability, allowing for better resource allocation and risk avoidance.
Real-world examples and case studies
Let’s talk about some real-world scenarios. Financial institutions have been using these models for years. Investment banks, for instance, tap into machine learning to evaluate client risk.
Remember Lehman Brothers in 2008? Before their downfall, red flags like high leverage and low liquidity ratios were waving. Some analysts pointed out that a solid model might’ve sounded louder alarms. Financial firms weave these models into their risk management systems.
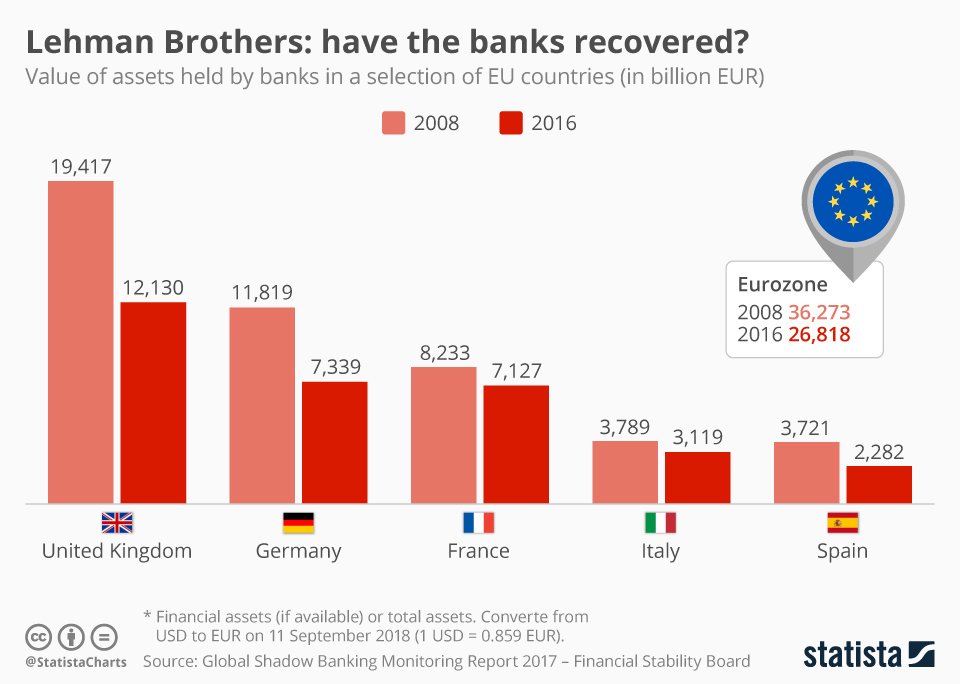
The results? Better risk assessments and timely interventions. These models are like having an early warning system. They help institutions steer clear of massive financial hits.
Key benefits
- Enhanced risk management: Identifies at-risk companies early for quick actions and strategy adjustments.
- Improved investment choices: Facilitates better investment decisions by avoiding high-risk investments.
- Resource allocation: Ensures resources are allocated efficiently to maximize benefits.
- Timely insights: Processes large amounts of data quickly, providing timely and actionable insights.
- Increased competitiveness: Keeps financial institutions agile and competitive in the market.
- Reduced losses: Helps minimize potential losses and bolster market stability.
#8. Tax evasion prediction
Tax evasion prediction is an intriguing application of machine learning in the financial world. Imagine having an eagle-eyed system that scans mountains of income and expense data, looking for anything unusual.
This technology is flipping the script for both financial institutions and tax authorities. Why? Because it automates the spotting of suspicious activities that might indicate someone is trying to dodge paying their taxes.
Real-world examples and case studies
Take the IRS in the United States, for instance. They’ve leveraged machine learning to sift through gigantic tax returns and financial transactions. The system throws up red flags for anything that seems fishy, streamlining the whole tax audit process. It’s like having a super-smart assistant who highlights the most suspicious files so you don’t have to wade through them all yourself.
Banks are on this train, too, using ML to monitor transaction patterns and report odd behaviours to tax authorities. Case studies are pretty straightforward—these systems drastically bump detection rates and reduce audit times. It’s fascinating to see how ML is reshaping financial oversight in such a practical way.
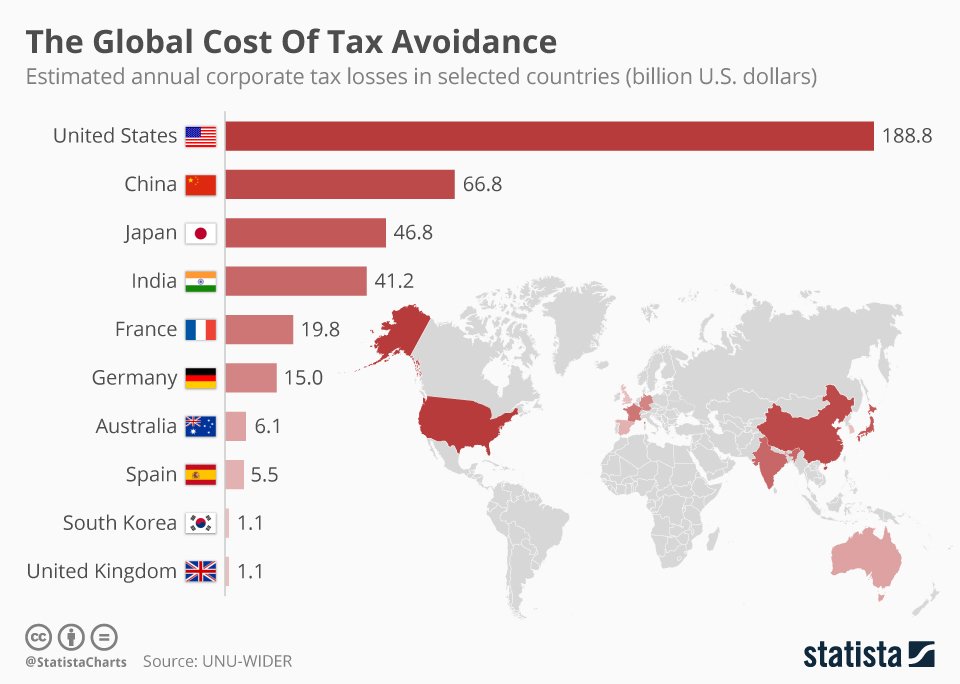
Key benefits
- High accuracy: Algorithms detect intricate patterns that indicate fraudulent activities, surpassing human capabilities.
- Resource optimisation: Automates initial detection, allowing tax authorities to focus on more complex cases.
- Efficient audits: Makes audits more efficient and effective by prioritizing high-risk cases.
- Data-driven insights: Provides valuable insights for planning and strategy.
- Enhanced audit quality: Improves the overall quality and effectiveness of financial audits.
- Considerations: Ensures systems are transparent and fair to avoid biases.
#9. Robo-advisors
How do robo-advisors work their magic in finance? These digital platforms, relying heavily on machine learning, automate financial planning with minimal human touch. They analyze your data—like your financial goals and risk tolerance—and offer tailored investment advice.
Think of them as your digital financial planners, crunching numbers to manage portfolios efficiently. This tech has revolutionised finance, making professional advice more accessible and affordable. It’s like having a savvy financial advisor in your pocket, democratizing investment management for all.
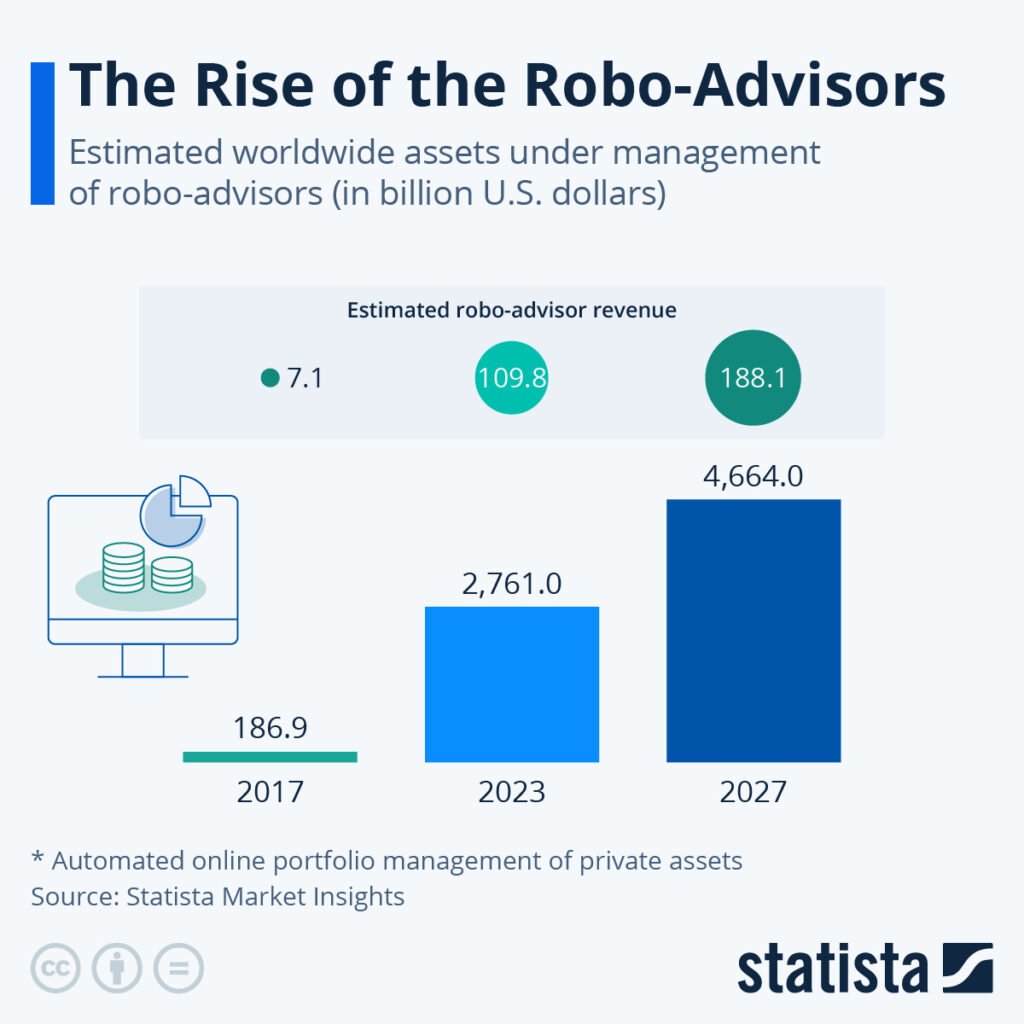
Examples and case studies
Take Betterment, for instance. This robo-advisor crafts personalized investment strategies and focuses on tax-efficient investing. It gathers user data and tailors advice to fit individual needs. Wealthfront is another big name associated with automated tax-loss harvesting.
And then there’s Charles Schwab’s Intelligent Portfolios, which leverages algorithms to balance and optimise your investments. These platforms aren’t just theoretical; they’ve shown real-world success by consistently delivering data-driven financial planning services. It’s like seeing artificial intelligence in action, making smart moves on your behalf.
Key benefits
- Cost-efficiency: Reduces the need for human labour, making financial advice affordable for smaller portfolios.
- Enhanced decision-making: Improves investment decisions and portfolio management by aligning investments with goals.
- Accuracy and efficiency: Algorithms provide precise and efficient financial advice.
- Address key challenges: Offers solutions to major issues in finance, making them valuable tools for modern investors.
- Considerations: While effective, they carry some risks and sometimes require human intervention.
#10. Financial monitoring
When discussing financial monitoring in machine learning, we dive into how algorithms monitor financial health like a vigilant watchman. By reviewing loads of data, these smart algorithms can detect patterns and anomalies faster than any human being ever could.
Needless to say, this tech is priceless for spotting risks and opportunities in real time. It integrates data, detects anomalies, and uses predictive analytics to foresee financial trends. In finance, this means better decision-making and risk management.
Real-world examples and case studies
Many big names in finance are already using machine learning to monitor and streamline financial operations. Take JPMorgan Chase, for instance. They use algorithms to sniff out fraudulent activities, such as a bloodhound on a scent trail. Then there’s Goldman Sachs, deploying machine learning to manage portfolios more effectively.
Integrating these machine learning models with existing systems might sound challenging, but the payoff is immense. These examples show how far-reaching and practical financial monitoring through machine learning can be, from catching fraudsters red-handed to fine-tuning investment strategies. It’s like having a financial guardian angel that keeps everything in check.
Key benefits
- Increased efficiency: Automates tedious, repetitive tasks, freeing time for strategic thinking.
- Enhanced accuracy: Real-time data analysis provides more reliable insights.
- Predictive analytics: Forecasts trends like seasoned market analysts.
- Improved fraud detection and risk management: Significantly enhances these critical areas.
- Better financial stability and growth: Contributes to institutions’ overall resilience and forward-looking capability.
- Considerations: While powerful, there’s a risk of over-reliance on automated systems.
#11. Customer service and chatbots
Customer service chatbots, driven by AI, are like the ever-reliable assistants in finance. Imagine having a virtual helper ready to answer your questions or help with transactions anytime.
In finance, these chatbots handle stuff like checking your balance, transferring money, and even giving you insights into your spending habits. They work by using natural language processing, so chatting with one can feel like talking to a real person.
Real-world examples and case studies
Take Erica, Bank of America’s chatbot, for instance. This AI marvel helps users check balances, move money around, and offer spending insights through a simple chat interface on their mobile app. The integration process was all about seamlessly embedding Erica into the app, creating a smooth, conversational experience. Users have raved about how Erica makes banking faster and easier.
Then there’s HSBC, using its chatbot to handle customer queries and offer global support.
Key benefits
- 24/7 availability: Ensures help is always accessible whenever needed.
- High interaction capacity: Handles numerous interactions simultaneously, reducing wait times.
- Efficiency: Frees up human agents to address more complex issues, enhancing overall customer service efficiency.
- Improved customer satisfaction: Boosts satisfaction by providing quick, accurate responses.
- Personalized interactions: Tailors’ responses to individual needs are crucial for delivering precise financial information.
- Operational efficiency: Significantly improves operational efficiency and customer contentment despite some limitations and nuanced issues.
#12. Regulatory compliance and reporting
Navigating regulatory compliance and reporting in finance? It’s no walk in the park. Banks and financial institutions face strict laws. Adhering to these rules isn’t just for show; it’s about honesty and managing risks. And if you’re wondering why they’re so strict? Well, it’s all to protect you, the customer.
They must accurately and timely report their operations, transactions, and financial data, akin to keeping a detailed logbook for regulators. This process ensures they stay within legal boundaries, prevent fraudulent activities, and maintain transparency.
Reporting involves not only documenting transactions but also analyzing data and generating comprehensive reports for regulatory bodies to prove compliance.
Real-world examples and case studies
JPMorgan Chase takes full advantage of machine learning to monitor transactions. They’re always on the lookout for anything fishy or out of the ordinary. The bank automates data collection, analysis, and reporting. This helps them flag potential fraud almost instantly. It’s like having a digital watchdog. These efforts help them adhere to anti-money laundering (AML) regulations.
Similarly, HSBC has dived into machine learning to identify anomalies and boost compliance efforts. These machine-learning systems sift through mountains of data, making the process more efficient and accurate. Just look at case studies showing how machine learning cuts down on manual work and reduces human error.
Financial giants like Bank of America also jumped on this tech bandwagon, streamlining their compliance processes. The results? Lower risks and better regulatory adherence.
Key benefits
- Enhances precision and speed: By automating those tedious, repetitive tasks, institutions can turn their attention to more strategic goals.
- Rapid adjustment: Quickly adapts to new regulations, keeping compliance in check and minimizing the risk of penalties.
- Decreases human error: This results to more accurate and reliable data.
- Faster fraud detection: Boosts the speed and effectiveness of recognising fraudulent activities.
- Enhanced risk management: Provides more effective tools when it comes to controlling and lowering risks.
- Cost reduction: Decreases operational costs through implementing automation, delivering smoother compliance processes.
- Industry-specific solutions: Targets distinctive issues, resulting to smarter decision-making and improved regulatory adherence.
- More resilient financial system: Builds a stronger and more robust financial infrastructure.
Challenges and solutions of implementing machine learning in finance
Let’s break down this journey into the following aspects: the challenges and the solutions we can employ.
Challenges
- Data quality: Getting high-quality, consistent data is like finding gold—it’s crucial for training effective machine learning models. Crummy data can mess up predictions, making AI apps unreliable. It’s like guessing the weather with broken instruments.
- Regulatory compliance: Trying to navigate the financial sector’s regulations like GDPR, AML and KYC? It’s a maze. Ever tried untangling Christmas lights? That’s what it feels like. As for non-compliance? Big fines and a damaged reputation are waiting for you.
- Skill gap: There’s a shortage of talent in AI and machine learning in banking. Finding those folks is like searching for a needle in a haystack. This lack of expertise makes it hard to implement these advanced technologies effectively.
Solutions
- Data management: Investing in solid data management systems is key. Banks must use solid data management frameworks, such as GDPR and BCBS 239. These will ensure their data stays accurate, consistent and secure.
- Compliance teams: Collaborating with compliance teams is critical to make sure that AI initiatives meet regulatory requirements. This means not just knowing the rules but baking compliance into AI model development. Basel III can be a helpful guide here.
- Training programs: To bridge the skill gap, banks should implement training programs focused on AI and machine learning. Upskilling current employees can help them use AI for fraud detection and risk management.
Key considerations for implementing machine learning in finance
- Start small: Kick things off with pilot projects. Show some value and get everyone on board before diving deeper.
- Ethical framework: Craft some solid guidelines for using AI responsibly. Consider potential biases, especially in financial decisions—nobody wants that mess.
- Explainability: Focus on models that people can understand. Transparency in financial processes is non-negotiable.
- Cross-functional collaboration: Team up across finance, IT, and data science departments. Success in this space requires everyone to pull together.
- Data governance: Lay down clear policies for collecting, storing, and using data. Good data governance is like the backbone of ML applications.
- Model lifecycle management: Set up processes to keep your models in check. Regular evaluations, retraining, and version control are critical.
Download your free “108 Gamification Elements and Mechanics”
Get your cheat sheet and have a quick reference at your fingertips!
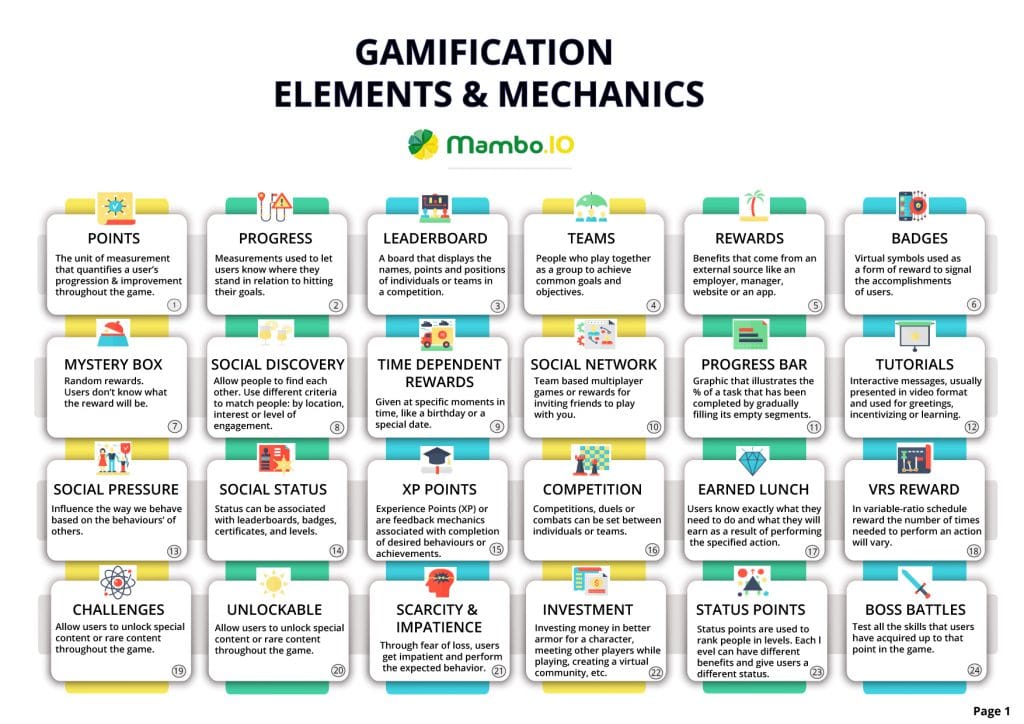
Conclusion
Machine learning in finance is shaking things up, right? It’s making everything faster, more accurate, and keeping customers happy.
Think about fraud detection – it’s like having a digital watchdog that never sleeps. Or consider risk management; it’s now sharper than ever. Sure, personalised financial services and algorithmic trading are getting pretty sophisticated, too.
Reality check on machine learning
But let’s not get too excited without looking at the whole picture. Data privacy issues are a big deal, no? And high computational demands? They’re like a sports car that needs constant refueling. Plus, finding skilled professionals is still a challenge.
Despite these bumps in the road, financial institutions really must embrace machine learning. Otherwise, they risk falling behind the curve. Staying competitive and driving innovation is key.
While implementing these technologies requires strategic investment, the benefits — cutting-edge, customer-centric services — are substantial.
The future of finance is closely tied to machine learning advancements, making adaptation essential for staying ahead. Whether institutions will fully embrace this change remains to be seen, but adapting is no longer optional.